Information gathering and research will continue to move at an accelerated pace toward real-time and dynamic. No one wants to read static information when more current and more accurate is possible and readily available. Delphi Method research can enjoy the best information dynamically as part of its decision-making process, while leveraging human expertise on a real-time basis: Human + Artificial Intelligence (H+AI). Eventually, machines (GenAI) will be able to make fuzzy decisions well, all by themselves, but for now we will want to leverage important decisions with well-integrated human intelligence.
Regenerative Dynamic articles are always more up to date than the information the author used in writing an article or blog. Hall and Lentz (in press) have been working on a H+AI project that creates Regenerative Dynamic articles and blog posts. The approach is to provide the queries or prompts given to GenAI engines so the reader can easily regenerate the responses at another time with the GenAI of their choice. The dynamic part of this is our encouragement to provide reliable sources including links to Wikipedia pages where relevant. Since Wikipedia is continually updated – especially on exponential growth topics like AI – the pages on the greatest crowdsource wiki of the world will be better at the time the reader links through than at the time the article was written.
So, in terms of Delphi Research, how does it change from the traditional Delphi to real-time research? And how might Delphi research be enhanced with AI? Feel free to use different GenAI engines. Often you will get more than one response with a little different theme. Some have more current information than others. We asked for tables and flow diagrams as well. A couple are attached with our “EDITOR NOTES” if we had any comments/thoughts.
Q: How might Delphi Research change from the traditional Delphi to real-time Delphi (or e-Delphi)? And how might Delphi research be enhanced with AI?
Q: Create a table showing Delphi Method in one column and possible AI Enhancements in another.
ChatGPT (2024, February).
Here’s a table comparing traditional aspects of the Delphi Method with possible AI enhancements:
Delphi Method | AI Enhancements |
Expert Selection based on facilitator’s knowledge | Automated expert identification and selection using AI algorithms analyzing publications and profiles |
Static Questionnaire Design | Dynamic and adaptive questionnaire design based on AI analysis of previous rounds |
Anonymity maintained manually | Enhanced anonymity and bias reduction through AI-managed processes |
Data Analysis through manual summarization and basic statistics | Advanced data analysis using NLP and machine learning to uncover patterns and insights |
Consensus reached through iterative rounds without predictive insights | Predictive modeling to forecast consensus likelihood and optimize the number of rounds |
This table showcases how AI can significantly enhance the efficiency, accuracy, and depth of analysis in the Delphi Method, making it a more powerful tool for reaching consensus among experts.
Q: Create a table showing traditional Delphi Method and possible AI Enhancements.
ChatGPT (2024, February) 2 / 2
Universal Primer
Here’s a table comparing the traditional Delphi Method with possible AI enhancements:
Aspect | Traditional Delphi Method | AI Enhancements |
Expert Selection | Manual selection based on facilitator knowledge and expert availability. | AI algorithms identify and select experts by analyzing large datasets of publications and profiles. |
Questionnaire Design | Standardized questionnaires, same for all rounds and participants. | Dynamic, adaptive questionnaires tailored by AI based on previous feedback. |
Anonymity & Bias Reduction | Anonymity maintained by facilitator; potential biases in summarization and feedback. | Enhanced through AI anonymization and bias detection algorithms to ensure unbiased feedback. |
Data Analysis | Manual analysis of qualitative and quantitative data; dependent on facilitator’s ability. | Advanced analysis using NLP and machine learning to uncover insights and patterns. |
Consensus Forecasting | Iterative process with no predictive analysis; relies on consensus emerging naturally. | Predictive models estimate likelihood of consensus, optimizing the number of rounds required. |
This table outlines how AI can enhance each aspect of the Delphi Method, from improving the efficiency and effectiveness of the expert selection process to optimizing the questionnaire design and analysis phases. AI integrations aim to streamline the Delphi process, reduce biases, and enhance the overall quality of the consensus reached.
Q: Create a flow diagram for the research process of a Delphi Method study.
Claude 2.0 (2024, April). Here is a flow diagram for the research process of a Delphi Method study:
… < rudimentary, text based flow diagram > …
EDITOR NOTE. Creating a flow diagram from various GenAIs was problematic. This version is rudimentary. If consensus is not reached, the flow goes nowhere! GenAI efforts to create a flow diagram that showed AI enhancements or real-time Delphi were unsuccessful. However, in a few months, or different AI tools, excellent results can be expected. Also, GenAI will happily describe what an infographic or a flow diagram might look like, which is a huge step toward creating it manually or with other (AI) tools.
Q: Based on the table by Hall & Jordan (2013), include columns on “Real-Time Delphi” and “Possible AI Enhancements”. Provide reliable sources and references including, where appropriate, active links to Wikipedia pages.
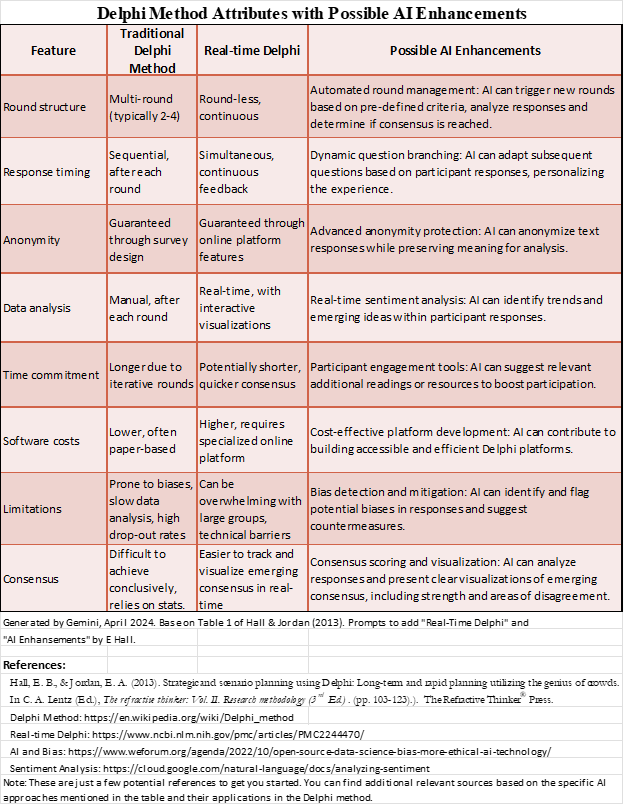
EDITOR NOTE. This table was adapted from Table 1 of Hall & Jordan by Gemini. Table exported to Excel for formatting, caption and reference.